Research
Machine Learning for Maritime and Coastal Security
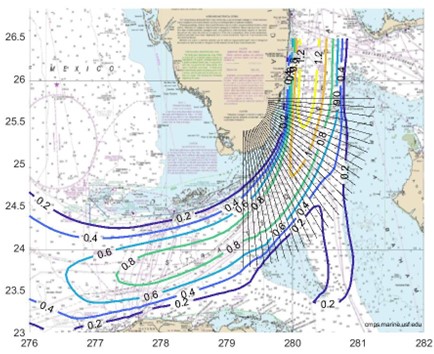
Figure 1. Contours of the mean current speeds around south Florida (m/s). Sampling lines used to compute frontal position are in black.
- Vessel identification
- Predicting hazardous currents
- Optimizing infrastructure
CMPS personnel are applying machine learning (ML) tools to help improve safety of
maritime operations. Vessel identification and description is critical to the safe
handling of ships as they move in and out of port as well as monitoring for illegal
activities at sea. This is typically done through the Automatic Identification System
(AIS). Large vessels periodically broadcast their information via AIS, but these data
are subject to instrumental and human error, as well as deliberate misrepresentation.
CMPS is working with the maritime community to develop new ML systems to detect and
correct AIS errors.
(more info here). Additionally, CMPS has developed a prototype ML system for predicting strong cross-currents
near Port Miami that can sweep ships into the walls of the navigation channel. The
system has been shown to provide greater than 90% accuracy for predicting the highest
cross-currents 12- and 24-hr in advance. (more info here). In a third project, CMPS personnel are working with the Tampa Bay Estuary Program
to develop and apply a genetic algorithm to help design modifications to the causeways
in Old Tampa Bay (OTB). Impermeable infrastructure can reduce the natural hydrodynamic
flushing of an estuary and is associated with reduced water quality. A series of cuts
through the three bridges spanning OTB are being considered to help restore the natural
circulation, but the number of possible location combinations is too large to fully
survey. The GA was designed to maximize flushing as represented in a numerical ocean
circulation model of OTB. The method is widely applicable to other locations dealing
with similar issues. (more info here)